What Metrics Should You Care About?
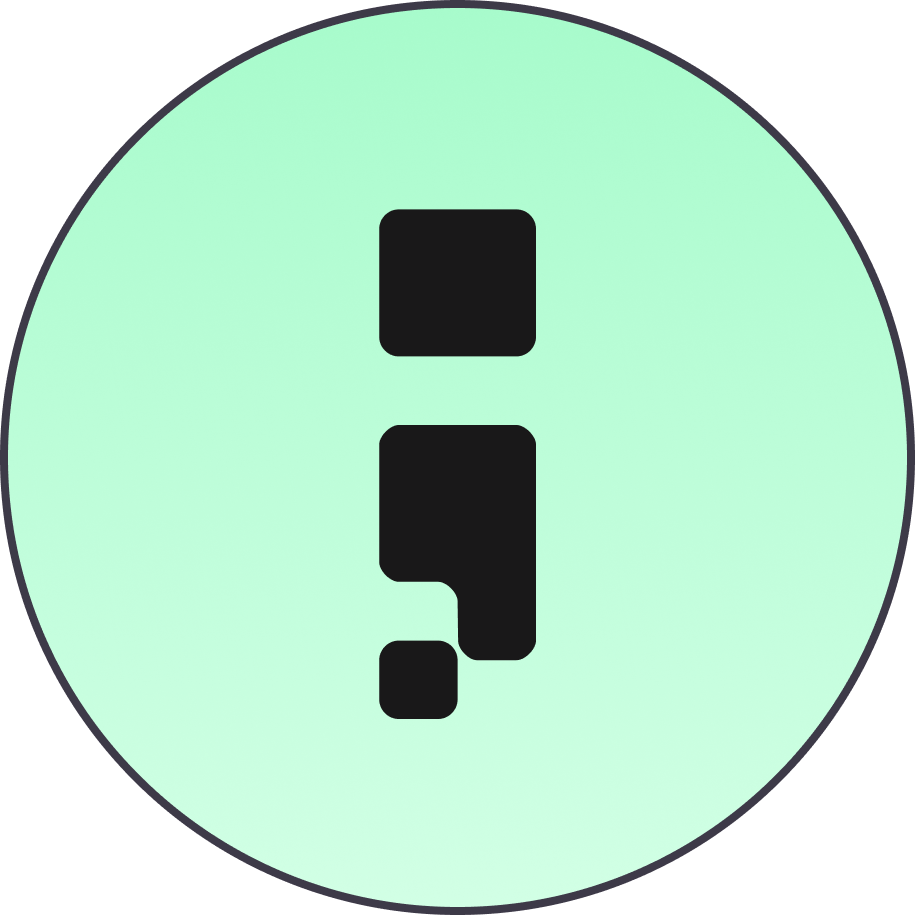

The path to creating clearly defined metrics from raw data can be a long and winding one. I’ve been through this process a few times within organizations at different stages of data maturity. I wanted to share my experiences in the hopes of making this process less painful for anyone working on it currently, or at minimum to make you feel less alone!
The first attempt: startups + early stage
My first experience trying to solve the “metrics problem” was at a 20-person B2B startup. My role was to help support the CEO and other department leaders with anything related to insights and analysis. This meant building a financial model forecasting our revenue and churn, creating a streamlined sales process in our CRM, or downloading a bunch of data to CSVs to analyze performance across the organization — a familiar story for many data teams of 1. At the time, there was very little written about how to calculate standard SaaS metrics. Whenever I managed to read something meaningful about best practices for KPIs, I’d immediately bookmark it.
The first couple months consisted of measuring what I would consider vanity metrics. These were mostly lagging indicators versus leading indicators, and it was never clear what decisions we could make based on the data we collected. We mostly leveraged internal reporting from third party tools: Woopra could tell us how many people were currently on the website, Google Analytics could tell us which pages had traffic, and Salesforce could tell us the number leads and opportunities we were generating. But none of these metrics gave us any insight into real performance or impact.
As we started to grow in number of customers we began the process of tracking standardized SaaS metrics. This was the first attempt to move past vanity metrics into the “measure what matters” zone.
The metrics we prioritized:
Churn (Revenue and Customer Count)
Churn helped us understand where and how we were losing customers and what we could do to get the right customers in from the beginning.
LTV (Lifetime Value)
LTV gave us insight into the long-term value of each subscription customer and how we could optimize our initiative to increasing this overall number.
CAC (Customer Acquisition Cost)
Measuring CAC informed our marketing team that we weren’t spending more than what we received from our customers. A good ratio of LTV to CAC is 3:1, which gave us a good benchmark we could work towards.
ACV (Annual Contract Value)
ACV told us the average revenue generated per year from a subscription account. In other words, it measured sales and marketing performance. Getting a deeper understanding of ACV helped us refine our target companies and increase this number over time.
Win Rates
Win Rates allowed us to get granular and pinpoint individual performance as well as zoom out and identify which acquisition channels or verticals were performing well.
At this stage, data still tends to raise more questions than it answers, but we were finally on our way towards metric maturity. This is the first big data milestone for most startups. They are able to create the first set of meaningful metrics that measure the overall health of the business. This lays the foundation for other teams to become more sophisticated as they grow.
The catch-22: Small, medium + midsize
Midsize companies are big enough to collect tons of data on their customers, but still small enough to only have the basics in terms of data infrastructure and resources. Most of the time you’re past the basics but still navigating your way to the next level.
When I started a new role at a much larger company (100+ employees), things were drastically different. They had already implemented a data warehouse, ETL tool, and even a BI/analytics tool.
One of the the most critical projects I worked on was building out a north star metric. ARR and revenue were important to measure, but they were still trailing indicators, and we wanted a metric that measured our customer experience more proactively. I did some analysis to assess what might be good indicators of customer success. Eventually, we were able to narrow down a metric we aligned on: power users per account. Of course, there was still definition work to be done. What did we mean by power user? How did we want to think about unique accounts?
Part of this analysis was also understanding what good vs. bad looks like. Eventually, we were able show that a threshold of at least 5 power users per account was correlated to a healthy customer. This became our north star metric. Every team and initiative became hyper focused on increasing this number either directly or indirectly.
This stage is generally where companies that have reached intermediate data maturity and are starting to move towards predictive data science and AI applications for data.
If you’re at this stage, you might be tracking the following types of metrics:
Product metrics
- Daily, Weekly, or Monthly Active users with some rolling windows like 7 day or 30 days.
- Average time spent in the product per account or number of events completed per user in an account.
Super metrics
- Complex metrics built on top of each other. An example would be number of power users per account — where a power user is defined as someone who has completed specific activities in the product or has some level of engagement.
- Customer Health Score — can be calculated from various data points, each assigned a different weight, to create an overall representation of customer health.
Enterprise: More data, more problems
Lastly, we have the very large enterprises.
I think most people assume that companies solve their data challenges once they reach a certain scale. In reality, large enterprises often struggle to manage large volumes of data across disparate systems and internal organizations.
Having consulted for these types of companies, I noticed while some part of the org had the best-in-class standards for metrics and reporting, other departments struggled with even the basics.
I remember working with a large public company that had been very successful in acquiring numerous companies over the years. However, each of their subsidiaries was calculating ARR and revenue metrics differently. Terms like bookings, accruals, billings, and revenue were thrown around, and in some cases, the metrics were slightly different, while in other cases, they were almost unrecognizable. As a result, we embarked on a multiyear project to help consolidate data across these subsidiaries, with the goal of tracking all their SaaS metrics consistently. It was complex and required input from many teams.
That’s where we come in
Creating reliable, informative metrics is hard at every stage. There are technical challenges, such as cleaning, transforming, and structuring data for analysis, and there are communication challenges. How do you get an entire organization aligned on what to measure and how to measure it from a technical perspectives?
This is the reason we built Preql. We aim to help solve the challenges of metric creation, maintenance, and access. Our experiences have taught us that measuring the right things will result in the desired business outcomes.
No matter what stage of your metric maturity journey you’re in, we would love to chat with you. Book a demo today.